The Center for Computational Research is planning a series of monthly talks on AI/ML techniques and applications across various scientific domains. We envision the talks to strike a good balance between depth and breath. The goal of these talks will be to (i) introduce the particular AI/ML technique to fellow faculty and graduate students who have a basic understanding of deep learning and (ii) present a variety of applications in different domains without assuming deep domain knowledge. Each event will start with a 45 minute talk with 15 minutes for questions and a subsequent 30 minute networking session for brainstorming and further discussion. The speakers will be URI faculty from a number of colleges.
Details on the first talk appear below.
Speaker: Peter Cornillon (GSO)
Date/Time/Location: May 1st, 3pm, 112 East Hall.
Title: Machine Learning Approaches for Characterizing Global Sea Surface Temperature Fields
Abstract: Sea surface temperature (SST) fields derived from satellite-borne sensors offer an ideal dataset for exploration using machine learning techniques. In this presentation, I will describe the use of an auto-encoder, in combination with a flow equalization technique, to identify outliers in a 20-year, global, twice-daily archive of SST fields. I will then demonstrate how this same approach can be applied to evaluate the performance of a global ocean circulation model.
Switching gears, I will introduce a machine learning model based on contrastive learning applied to the same SST datasets—this time to uncover and categorize fundamental spatial patterns within the fields. Finally, I will briefly touch on an analysis of the latent space produced by the contrastive learning model, with a focus on estimating the intrinsic dimensionality of SST field variability.
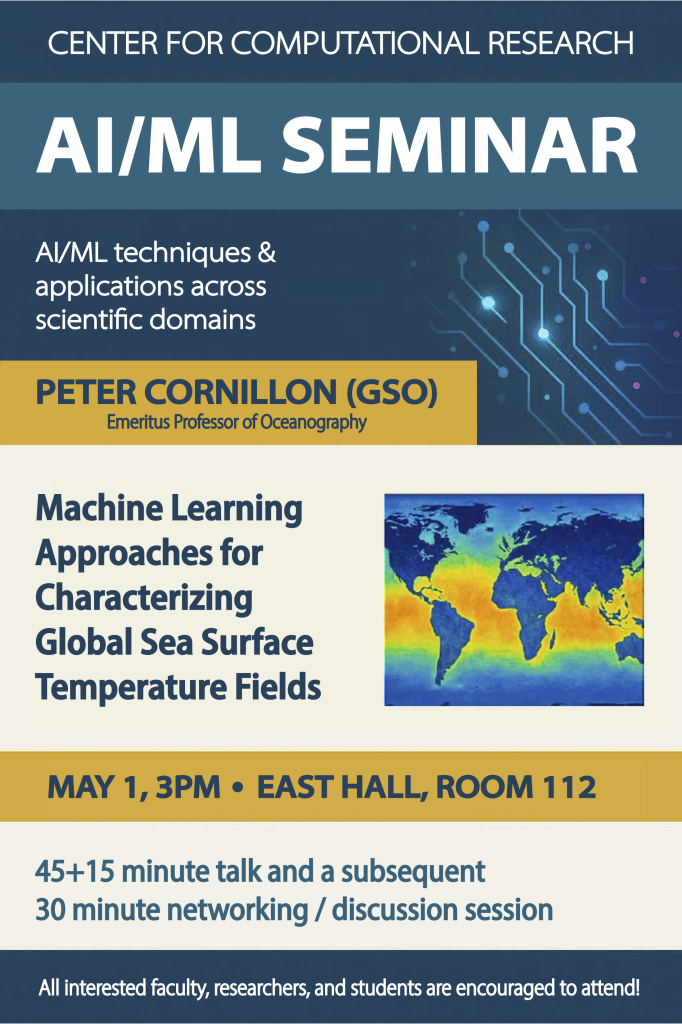